Image Captioning menurut Scientific Revolution Kuhn dan Popper
Abstract
Image captioning is one area in artificial intelligence that elaborates between computer vision and natural language processing. The focus on this process is an architecture neural network that includes many layers to solve the identification object on the image and give the caption. This architecture has a task to display the caption from object detection on one image. This paper explains about the connection between scientific revolution and image captioning. We have conducted the methodology by Kuhn's scientific revolution and relate to Popper's philosophy of science. The result of this paper is that an image captioning is truly science because many improvements from many researchers to find an effective method on the deep learning process. On the philosophy of science, if the phenomena can be falsified, then an image captioning is the science.
References
R. K. Srihari, “Use of Captions and Other Collateral Text in Understanding Photos,†1994, pp. 1–32.
J. Gu, G. Wang, J. Cai, and T. Chen, “An Empirical Study of Language CNN for Image Captioning,†in IEEE International Conference on Computer Vision An, 2017, pp. 1231–1240.
Y. U. and T. H. Andrew Shin, “Image Captioning with Sentiment Terms via Weakly-Supervised Sentiment Dataset,†in British Machine Vision Conference, 2016, p. 53.1-53.1.
J. Aneja and A. G. Schwing, “Convolutional Image Captioning.â€
B. Dai, “Contrastive Learning for Image Captioning,†in Advances in Neural Information Processing Systems Conferece, 2017, no. 30, pp. 898–907.
K. Shuster, S. Humeau, H. Hu, A. Bordes, and J. Weston, “Engaging Image Captioning via Personality,†in IEEE Conference on Computer Vision and Pattern Recognition, 2019, pp. 12516–12526.
B. Dai and S. Fidler, “A Neural Compositional Paradigm for Image Captioning,†no. NeurIPS, pp. 1–11, 2018.
K. Papineni, S. Roukos, T. Ward, and Z. Wei-Jing, “BLEU: a Method for Automatic Evaluation of Machine Translation,†in Proceedings of the 40th Annual Meeting of the Association for Computational Linguistics (ACL), 2002, pp. 311–318.
J. Devlin et al., “Language Models for Image Captioning : The Quirks and What Works,†Comput. Lang., pp. 100–105, 2015.
V. Kougia, J. Pavlopoulos, and I. Androutsopoulos, “A Survey on Biomedical Image Captioning,†2016.
X. Li, X. Song, L. Herranz, Y. Zhu, and S. Jiang, “Image Captioning with both Object and Scene Information,†in 24th ACM international conference on Multimedia, 2016, pp. 1107–1110.
D. Shin and I. Kim, “Deep Image Understanding Using Multilayered Contexts,†Math. Probl. Eng., vol. 2018, pp. 1–11, 2018.
Z. Gan et al., “Semantic compositional networks for visual captioning,†Proc. - 30th IEEE Conf. Comput. Vis. Pattern Recognition, CVPR 2017, vol. 2017–Janua, pp. 1141–1150, 2017.
A. Karpathy and L. Fei-Fei, “Deep Visual-Semantic Alignments for Generating Image Descriptions,†IEEE Trans. Pattern Anal. Mach. Intell., vol. 39, no. 4, pp. 664–676, 2017.
X. He, B. Shi, X. Bai, G. Xia, and Z. Zhang, “Image Caption Generation with Part of Speech Guidance,†Pattern Recognit. Lett., vol. 0, pp. 1–9, 2017.
J. Mun, L. Yang, Z. Ren, N. Xu, B. Han, and A. Go, “Streamlined Dense Video Captioning.â€
J. Aneja and A. G. Schwing, “Convolutional Image Captioning,†Comput. Vis. Pattern Recognit., pp. 5561–5570, 2017.
G. Ding, M. Chen, S. Zhao, H. Chen, and J. Han, “Neural Image Caption Generation with Weighted Training and Reference,†Cognit. Comput., p. 10.1007, 2018.
C. Gan, Z. Gan, X. He, and J. Gao, “StyleNet : Generating Attractive Visual Captions with Styles,†in IEEE Conference on Computer Vision and Pattern Recognition (CVPR), 2017, pp. 955–964.
S. Bai and S. An, “A survey on automatic image caption generation Shuang,†Neurocomputing, vol. 311, pp. 291–304, 2018.
T. Kuhn, The Structure of Scientific Revolutions, 4 (2012). University of Chicago Press, 1962.
D. Mahayana, Filsafat Ilmu Pengetahuan. Bandung, Indonesia: ITB Press, 2018.
J. Deng, W. Dong, R. Socher, L. Li, K. Li, and L. Fei-fei, “ImageNet : A Large-Scale Hierarchical Image Database,†in IEEE Conference on Computer Vision and Pattern Recognition (CVPR), 2009, pp. 248–255.
“F. Serafino, G. Pio and M. Ceci, ‘Ensemble Learning for Multi-Type Classification in Heterogeneous Networks,’ in,†vol. 30, no. 12, p. 8525379, 2018.
H. Kaiming, Z. Xiangyu, R. Shaoqing, and S. Jian, “Deep Residual Learning for Image Recognition,†Comput. Vis., pp. 1–9, 2016.
A. Krizhevsky, I. Sutskever, and G. E. Hinton, “ImageNet Classification with Deep Convolutional Neural Networks,†Adv. Neural Inf. Process. Syst. 25 (NIPS 2012), vol. 25, pp. 1–9, 2012.
Y. Lecun, L. Bottou, Y. Bengio, and P. Haffner, “Gradient-Based Learning Applied to Document Recognition,†in IEEE, 1998.
K. Simonyan and A. Zisserman, “Very Deep Convolutional Networks for Large-Scale Image Recognition,†arxiv, pp. 1–14, 2015.
J. Donahue et al., “Long-term Recurrent Convolutional Networks for Visual Recognition and Description,†pp. 1–14, 2015.
V. Kougia, J. Pavlopoulos, and I. Androutsopoulos, “A Survey on Biomedical Image Captioning,†in Proceedings of the Second Workshop on Shortcomings in Vision and Langua, 2016, pp. 26–36.
H. Agrawal et al., “nocaps : novel object captioning at scale,†in IEEE International Conference on Computer Vision (ICCV) 2019, 2019.
V. Batra, Y. He, and G. Vogiatzis, “Neural Caption Generation for News Images,†pp. 1726–1733, 2016.
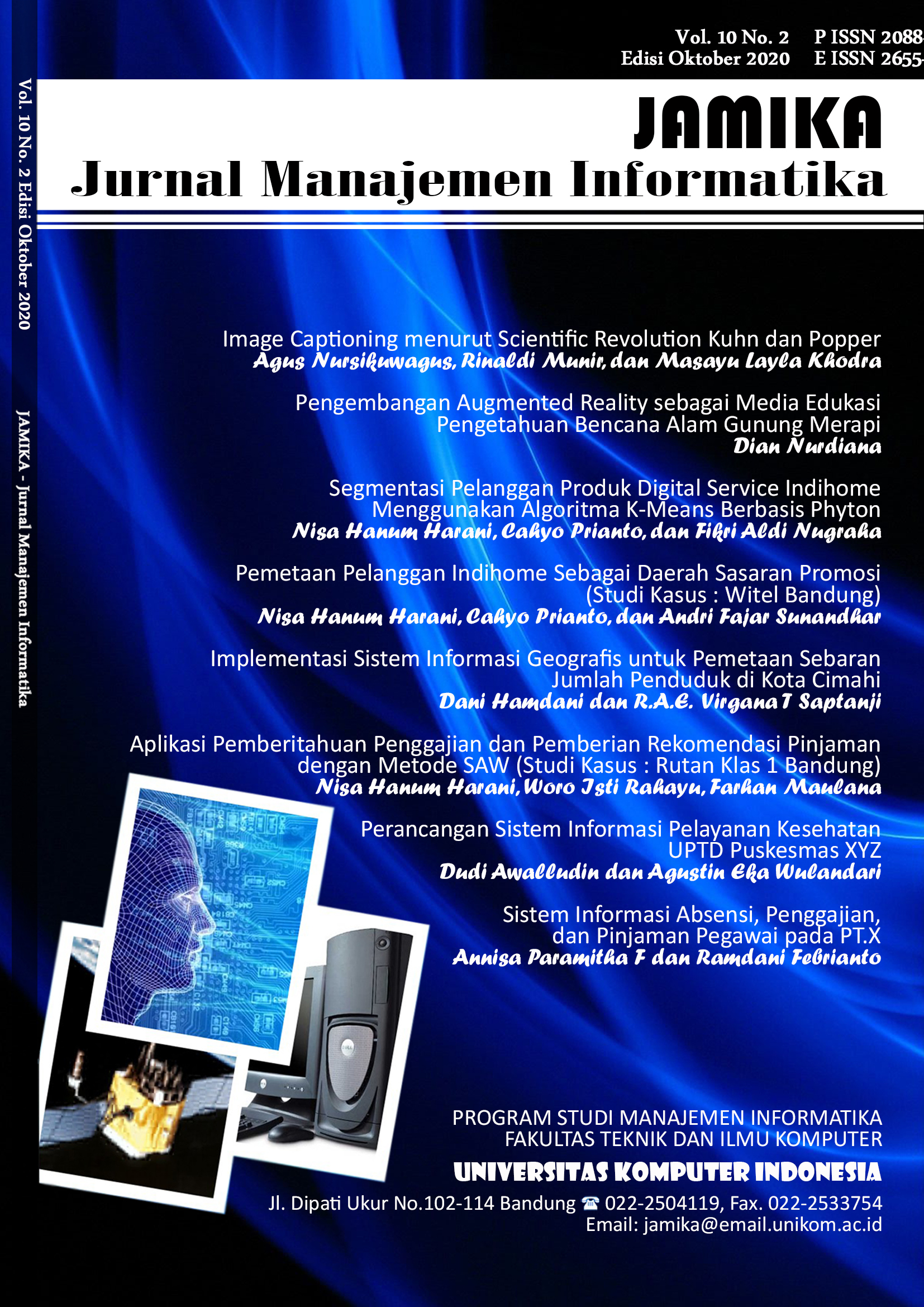